AI and neuroscience
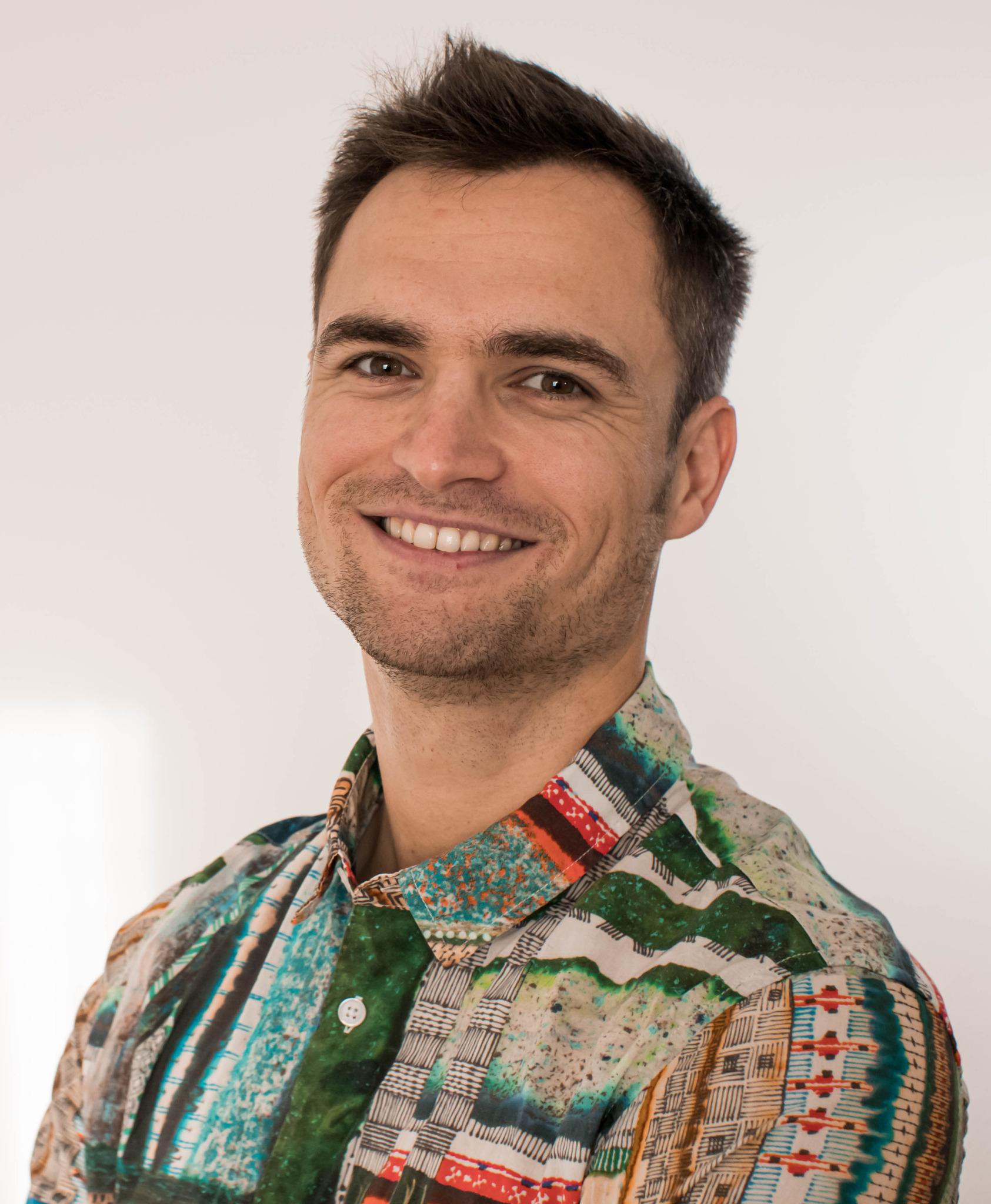
We live in exciting times: On the one hand, artificial intelligence (AI) is revolutionising data science, while on the other, new recording techniques are able to record brain activity with increasing accuracy. In our research group, we focus on the connection between AI and brain research. We draw on our knowledge of theoretical computer science and practical AI development and work closely with neuroscientists. Our group pursues two main goals: Firstly, we use AI and deep learning to develop new methods for analysing brain data. Secondly, we are researching how the brain works in order to develop new AI systems that are inspired by the brain.
With AI, we want to decode the functioning of the brain using electrophysiological recordings. These pioneering recordings use fine electrodes that can record the activity of individual nerve cells very precisely. We are currently working with recordings from the mouse brain in order to understand the basic processes in the animal brain. Our ambitious goal is to enable a better understanding of the physical laws and mechanisms of the brain by combining data-driven modelling of the brain with basic principles of brain computation. Imagine a future where models like ChatGPT can analyse brain images in hospitals. We want to ensure that the model interprets the recorded activity physically correctly and provides helpful clinical information.
In the other direction, we use our observations from the recordings to develop new computer algorithms. The brain has inspired the development of AI many times, and we believe it can also help us build more energy-efficient AI computers. Specifically, the brain communicates through short electrical impulses called spikes. These spikes often occur in dense packets that are synchronised with surprising and unpredictable events. We suspect that this hides a fundamental principle of information transfer in the brain, which we can describe with the help of machine learning theory. We are developing new algorithms using this type of event-based predictive coding. In audio compression, for example, we can convert a sound recording with customised AI models into spike packets, whereby the transmission of the spikes only requires a few bytes. This kind of bandwidth saving could be crucial today, as our computer architectures consume most of the energy for data transfer. Together with our partners who are developing new experimental AI chips, we see real potential to develop more energy-efficient chips for tomorrow's world.