Understanding Language in Context
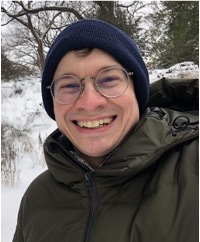
AI assistants such as ChatGPT or Bard have demonstrated the potential of automated language processing over the past year. In many cases, such assistants can help with brainstorming, compose simple texts or automatically generate program code, among other things. These assistants are based on so-called Large Language Models (LLMs), which generate an answer word by word based on an input.
Thanks to major leaps in development in recent years, LLMs and AI assistants based on them can now often understand complex questions and generate suitable answers. However, if we move away from individual examples and subject LLMs to a more systematic analysis, it turns out that the answers generated by LLMs are still unreliable in many cases. For example, current systems fail to answer questions about long texts correctly or to read between the lines.
Thus the overall goal of this research project is to improve the comprehension capabilities of LLMs. In this context, we will develop new models that are better suited to process long texts and understand indirect speech. A specific goal is to combine current research in machine learning and cognitive science to develop new architectures for neural networks that can reliably identify the entities (e.g. people, organizations, objects, etc.) in a text and their relationships, which is a prerequisite for understanding long texts. To evaluate our and other models more systematically, we will also develop new evaluation methods, such as benchmarks that test the reading comprehension of LLMs. During development, we will place a special focus on ensuring that models cannot take "shortcuts" when answering questions and thus only appear to understand texts. This will help us to better understand what capabilities and limitations current and future language models actually have and how they can be improved. All of this has the potential to form the basis for new AI systems that can analyze large amounts of text (e.g. collections of biomedical articles) in depth and answer complex questions.