Building Robust and Explainable AI-based Defenses for Computer Security (BREADS)
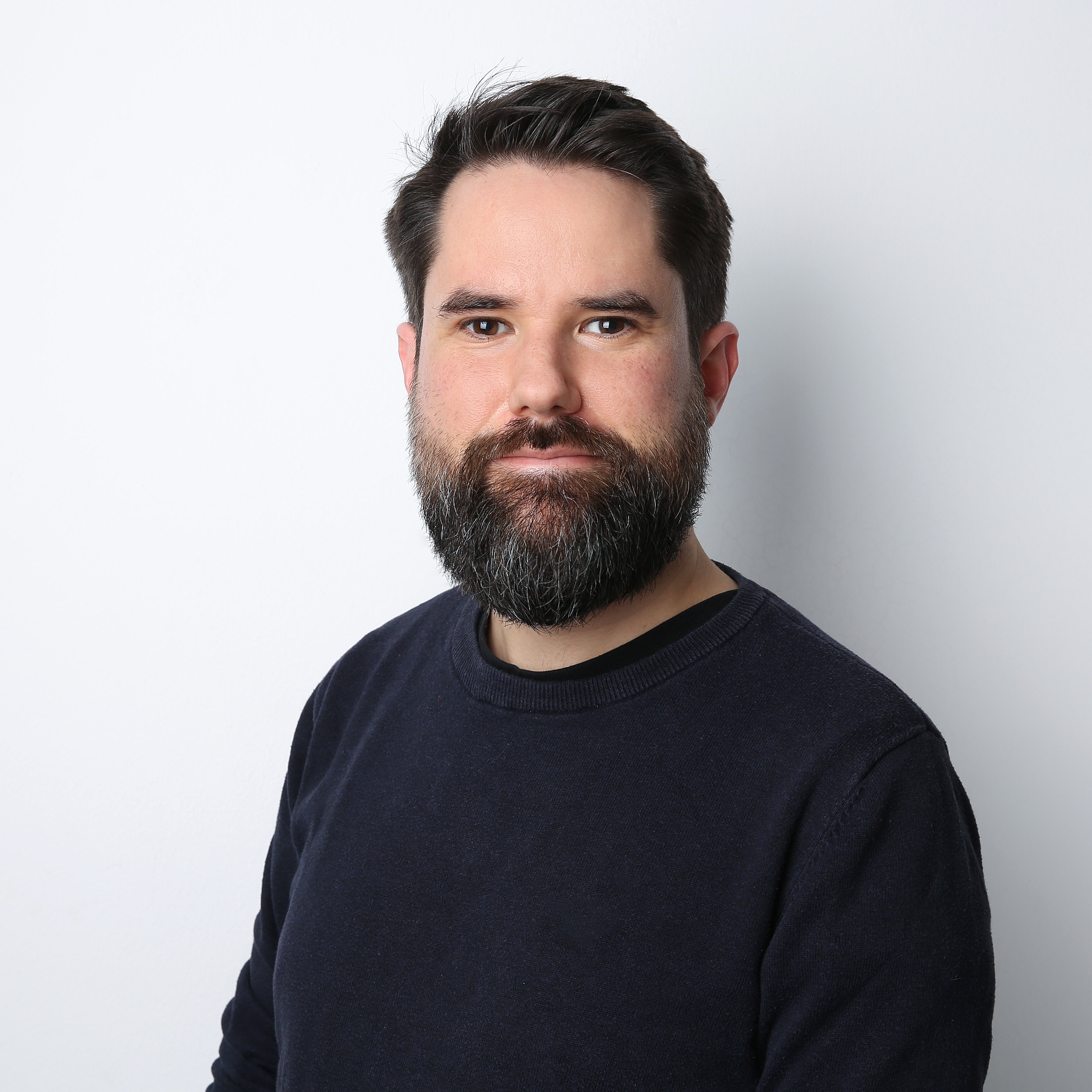
No day goes by without news of severe cyber attacks against companies, public facilities, and critical infrastructure—including even hospitals and power plants. Although the incentives of malicious actors that conduct these attacks are manifold, the vast majority are primarily perpetrated to gain financial profit. As a result, an extensive network of professional, fraudulent actors has emerged in recent years, leading to a wide variety of malicious activities with which companies and law enforcement agencies must contend on a daily basis. Traditional defenses, like signature-based systems for spam and intrusion detection, require manual effort to update their detection patterns. Thus, they are unsuitable for coping with the large number of fast-evolving malicious activities that emerge every day. To counter this threat, machine learning-based techniques have been extensively explored, as these methods extract effective detection patterns from large amounts of data automatically. Although promising, these learning-based detection methods suffer from severe drawbacks that limit their applicability in real-world settings. First of all, the underlying models are often very complex and their decision-making process is hard to interpret even for human analysts with expert knowledge. Secondly, learning-based systems have shown to perform poorly if train and test distributions differ, a phenomenon known as dataset shift that affects many security domains. As a result, these systems either fail completely in real-world settings or become outdated quickly, requiring continuous retraining of the underlying machine learning models, which is time-consuming and computationally expensive. Even worse, very recently, it has been found that the state-of-the-art learning model ChatGPT itself has already been used by malicious actors to generate new frauds automatically, thereby potentially transforming the way fraudsters operate. Consequently, the impressive capabilities of recent learning-based models raise serious concerns regarding their potential misuse by malicious actors, as these learning models will change the threat landscape in the upcoming years and likely speed-up the evolution of new fraud schemes even further. To keep pace with the fast-evolving threat landscape, we aim to address three key security research questions throughout this project. In particular, we want to better understand the decision-making process of current learning-based methods in security and to use this knowledge to improve their robustness in the presence of dataset shift. Finally, we use the developed methods to understand and adequately address the rising threat introduced by AI-generated fraud. The project is divided into three parts: 1. In the first part of this project, we seek to overcome the limitations of current explanation methods by developing new methods that allow us to better understand the decision-making process of learning models in security. To this end, we aim for novel techniques that highlight high-level concepts relevant to the prediction of a learning model. Using these techniques, we can gain insight into why the performance of current models decreases and how to address their weaknesses. 2. In the second part, we plan to develop new learning-based methods to cope with different types of dataset shifts in various security domains, such as malware detection and vulnerability discovery. Here, we guide our research along the following two dimensions: First, we want to develop new deep learning-based techniques that operate by design on novel, robust feature spaces that encode semantic information, thus being more robust in the face of dataset shift. Second, we plan to examine alternative strategies to address dataset shifts, such as methods from the active learning field. 3. Finally, in the third part of this project, we use the previously developed techniques to analyze and address a fast-evolving security threat that will change the future threat landscape: The rise of AI-generated fraud, such as deep-fake images and videos, misleading information, and even sophisticated malware. The speed and scale of such developments in recent years make it likely that this novel threat will become one of the biggest challenges for security researchers. Equipped with the new techniques developed throughout this project, however, we aim to be able to tackle these threats as they emerge.