An interdisciplinary approach to learn and test the causal mapping between neural network dynamics and behavior
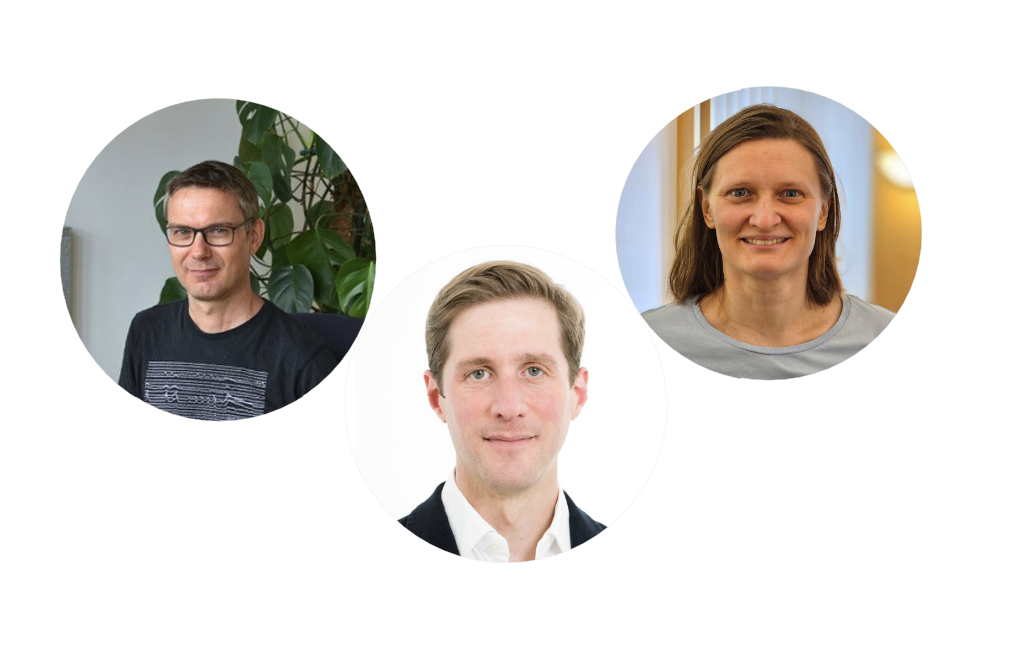
Studies across various species, like invertebrates and mammals, including primates, have shown that behavior can be represented by coordinated activity patterns among large neuronal populations in relevant brain areas. However, the underlying control mechanisms that dictate how the brain makes behavioral decisions by choosing and switching between alternative network activity states are poorly understood. To address this problem, we here propose to build an interdisciplinary team of machine learning experts, computational neuroscientists, and experimentalists and leverage the tractable model organism C. elegans. Novel machine learning approaches will be applied to make causal inferences from C. elegans whole brain activity data and in silico simulations of its neuronal network. The resulting hypotheses and predictions will be tested through optogenetic interrogations during single cell resolution whole brain functional imaging. An innovative artificial intelligence (AI) approach will be developed to infer brain states in real-time, enabling closed-loop optogenetic control. These studies aim to uncover fundamental principles underlying the control mechanisms of neuronal network activity and behavior, which are relevant to all animal brains.