Determinants of mRNA Lifetime and Translation Efficiency
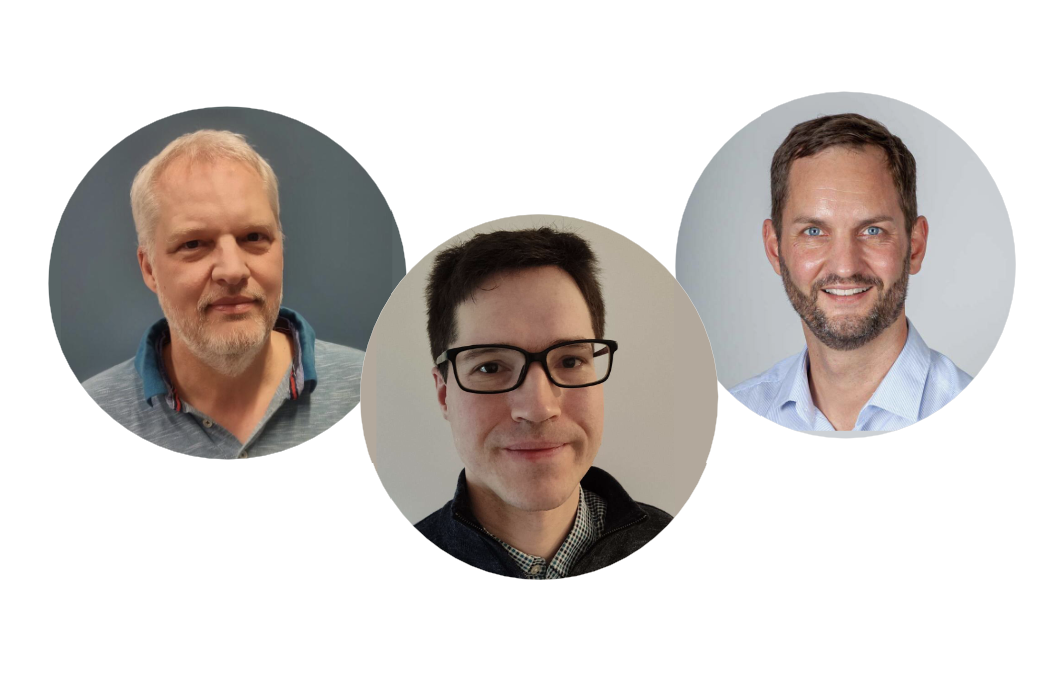
Eukaryotic cells employ a variety of regulatory mechanisms to precisely control protein production. Many of these mechanisms act on the level of the messenger RNA via features encoded in the mRNA itself. However, only a few of these features have been well studied. RNA structure is likely to influence both translation and RNA decay. However, while stable secondary structure protects RNA from hydrolytic decay, some structures may hinder translation, as is well known for translation initiation in prokaryotes.
In this project, we will employ deep learning, to discover RNA features relevant for translation and lifetime in an unbiased way. We will train models to predict properties such as decay rates, ribosome occupancy, and protein expression, from the sequence as well as additional annotations, such as UTRs and coding region. In addition, the input will include RNA secondary structure information, such as accessibility and the location of locally stable structures. The trained models will be analyzed to discover the most relevant (groups of) features and ultimately the underlying biological mechanisms. Hypotheses will be tested by measuring RNA decay and translation on sequences specifically designed to exhibit certain sequence/structure properties.
While the predictors will be of immediate interest for optimizing synthetic mRNAs in medical and biotechnological applications, the focus of the project will be on elucidating mRNA features and the underlying biological mechanisms that control protein production.