mlDIAMANT: machine learning decodes interaction archetypes of membrane proteins to predict the effect of genetic variants
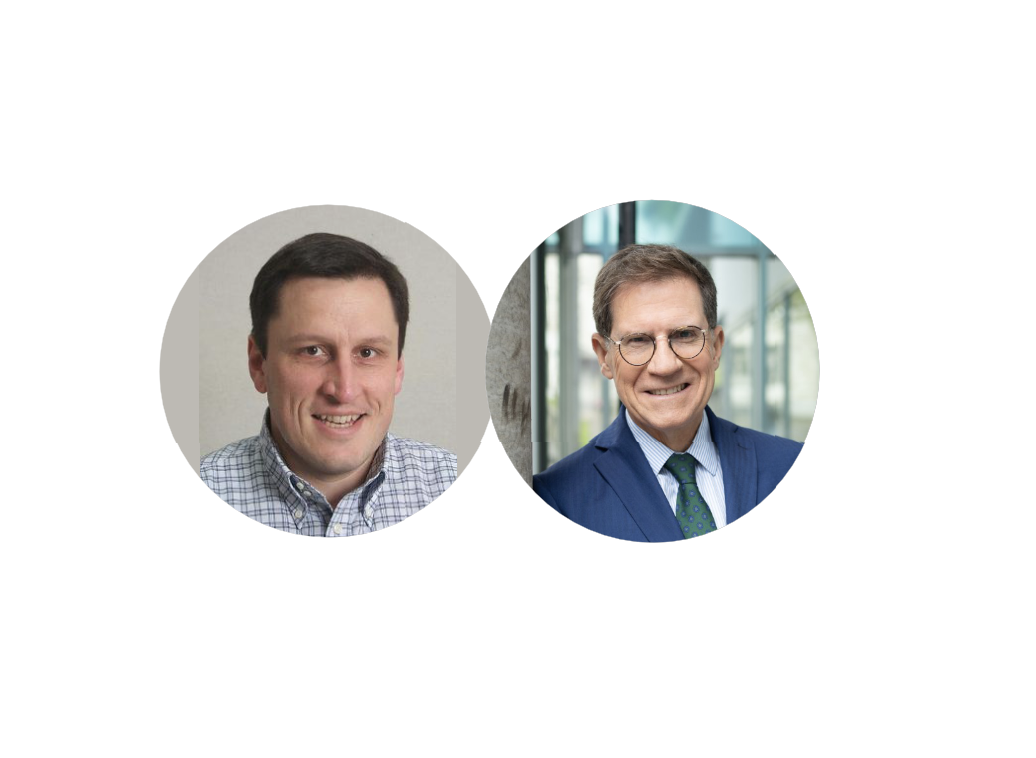
Machine learning can empower scientific advances in areas of biology which are of fundamental importance, yet difficult to achieve with standard approaches. Even though proteins need to interact with other proteins to achieve biologically meaningful function, the "code" determining their interactions has not been fully deciphered. As a first integral contribution in cracking this code, we propose to use machine learning to break down the problem into a manageable number of interaction types, which we call "archetypes". Motivated by their prominent role in cell communication and disease, we propose to focus on interactions of membrane proteins. Our goal is to build a structural catalog of protein-protein interaction (PPI) archetypes and use it to predict the effect of human genetic variation. We are in the unique position of leveraging more than 2,000 unpublished, but open access, affinity-purification mass spectrometry experiments carried out in our laboratory as part of another discovery campaign. Armed with this unique physical interaction data, we use artificial intelligence to build structural models of PPIs and machine learning to classify modes of interactions based on sequence and structure features. Many disease-associated genetic variants affect membrane proteins and are thought to affect PPIs. The derived "archetypes" will serve as a grammar for membrane PPIs and will be experimentally tested for their ability to predict the effect of genetic variants segregating in human populations.