Understanding pancreas biology with AI/ML
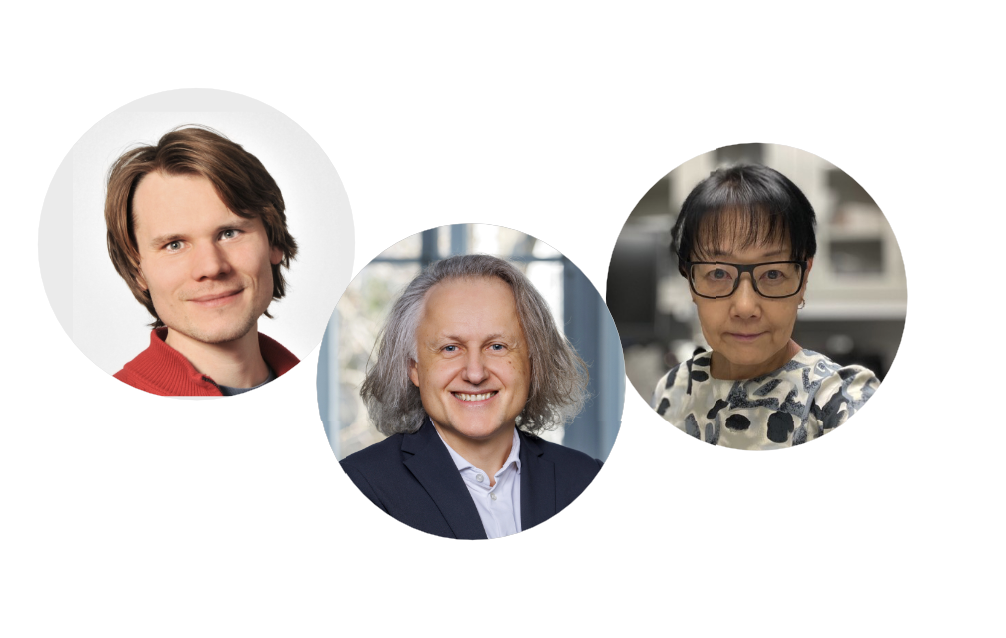
Manami Hara (The University of Chicago)
This project will use biophysically-constrained machine learning (ML) to address functional and medically-relevant questions in pancreas sensory function. We will analyze existing (and acquire new) functional and morphological datasets of pancreas cell collectives and their relationship to the perfusion of the organ. Pancreas is fascinating because cells in its collectives must act jointly, in coordination, to sense the metabolic state of the body and respond precisely by appropriate hormone secretion; breakdowns in these processes can result in diabetes or pancreatitis. While substantial effort has been invested during past decades at the sub-cellular level to understand molecules and pathways within individual cells, how those cells interact to achieve reliable sensory/response function has remained unclear. We will use simultaneously recorded activity of many cells in pancreatic slices at unprecendented temporal resolution and stimulus control to infer these interactions. Methodologically, we not only aim to apply out-of-the-box ML technology for image processing and disease prediction, but principally to develop novel ML approaches in the unsupervised and reinforcement-learning domain to extract interpretative and normative models of the cell collective function, thereby iteratively driving new experiments. Specifically, we aim to identify subpopulations of different cell types online (supervised learning); to extract cell-cell interactions and model their joint activity distribution in defined physiological and diet states, under pharmacological perturbations, and in disease (unsupervised learning); and to predict optimal cell collective function under time-varying naturalistic metabolic conditions (reinforcement learning). This interdisciplinary theory-experiment collaboration has the potential to significantly advance our understanding of the biology and pathology of the pancreas in particular, as well as of chemical signaling in cell collectives in general.