A Digital Well-Being Index for Vienna - Extracting Regional Indicators of Subjective Well-Being from Digital Content Streams
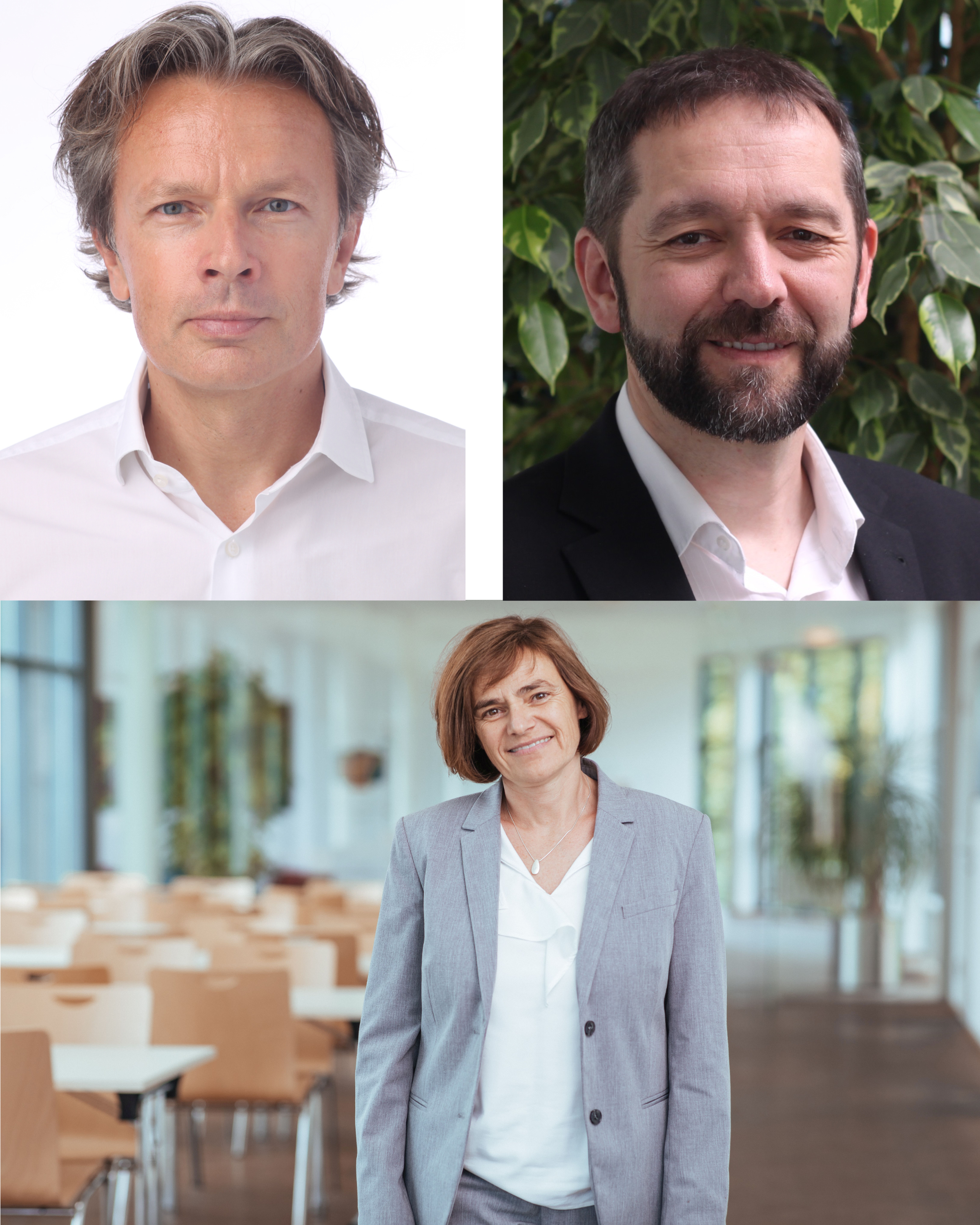
Vienna has been voted Best City for Quality of Life for many years. How can stakeholders get insights into perceived well-being in a timely manner and related to specific aspects of urban environments? Comprehensive national surveys are costly, require significant organizational effort and are a careful balance between knowledge to be gained and burden placed on respondents. They take a lot of time to compile until official results can be published, and often lack regional information since predefined questions only allow for limited customization. The rather generic nature of certain well-being metrics (e.g. “satisfied with life”) have constrained the usefulness of academic results to support policy decisions. To address these challenges and provide insights into subjective well-being and related social issues, we propose AI-based natural language processing in conjunction with evolving knowledge graphs to extract affective knowledge from user-generated digital content. Automatically mapped to existing indicators, this knowledge will complement and enrich survey-based approaches and traditional assessment methodologies, mitigating some of the associated problems. Computational approaches based on large-scale online data provide a near real-time analysis of evolving citizen perceptions. Most previous approaches have focused on specific topics, however, and lack the scope required for a systematic comparison to enrich existing indicators of national and regional well-being.