Communication and Complexity Constrained Inference over Graphs for Big Data
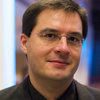
The main objective of the project Co3-iGraB as stated in the grant proposal was to “devise and analyze efficient decentralized inference methods for big data problems by combining the emerging field of graph signal processing with stochastic optimization methods, thereby achieving a flexible trade-off between computational and communication resources on the one hand and inferential power, i.e., estimation accuracy, on the other hand.” The specific research topics we focused on in the course of the project included the design of sampling and reconstruction algorithms for signals on graphs, the problem of learning the graph topology from given data, the machine learning task of data clustering, and the fundamentals of the new concept of signed graph signal processing.
The project team consisted of the Principal Investigator Prof. Matz and several Post-Docs and Ph.D. students at Vienna University of Technology (VUT) and project partners Prof. Alex Jung (Aalto Univ., Finland), Prof. Yonina Eldar (Technion, Israel), Prof. Mike Davies (Univ. Edinburgh, UK), and Prof. Pablo Piantanida (CentraleSupélec, France). The scientific results of the work performed in the course of the project essentially lay the foundations for solving important graph signal processing tasks using the novel concept of graph total variation. Graph total variation is a metric for quantifying the smoothness of graph signals that essentially promotes sparsity on the signal’s graph gradient. Using this metric, we devised algorithms for reconstructing missing data (signal inpainting) and studied their performance (cooperation with Technion), for identifying the interaction patterns of items in large data sets (graph learning), and for splitting data sets into homogeneous groups of similar items (clustering). We further advanced the understanding of the performance of algorithms for sparse recovery (collaboration with the University of Edinburgh), devised interpolation methods for signals sampled in oblique subspaces (together with the University Vienna), and developed information-theoretic foundations for data science problems in terms of the information bottleneck (collaboration with CentraleSupélec).
The projected results lead to the publication of 10 articles in top journals, 12 papers at flagship conferences, and the completion of 4 PhD thesis. We further disseminated our results via a full-day course at the Summer School on Complex Networked and Control Systems, organized by the research center Dependable Internet of Things of TU Graz in July 2018